As performance gaps narrow and model costs drop, the open vs. closed model debate is in the spotlight. This brief explores enterprise adoption in the landscape and the cost dynamics reshaping the divide.
This is part 2 in our series on the generative AI divide. In part 1, we cover the open-source vs. closed-source foundation model landscape.
Open-source AI is drawing unprecedented attention from developers and enterprises, driven in part by DeepSeek’s recent model releases.
Cost pressures and demands to improve the performance of generative AI applications are driving enterprise interest in the ecosystem as organizations seek more flexible and cost-effective alternatives to proprietary solutions.
Meanwhile, performance gaps have been closing between leading closed-source and open-source models, highlighted by Meta’s Llama model family.
Note: For the purposes of this analysis, we consider “open-weight” models (like Llama) to be on the “open-source” model spectrum. Graphic excludes reasoning models.
Below, we use CB Insights data to dive into how enterprises are choosing between models, the supporting vendor ecosystem, and the economics reshaping the open vs. closed-source divide.
Hybrid model approach dominates enterprise adoption: Based on CB Insights’ 30+ customer interviews, organizations are prioritizing experimentation with multiple models, with 94% using 2 or more LLM providers. This hybrid strategy — often across open and closed models — allows companies to optimize for specific use cases, balancing performance, control, and cost considerations while reducing dependency on single providers.
Cloud providers are key distribution channels for both open and closed models: Cloud platforms are critical infrastructure partners for the genAI era, both through direct model serving and by enabling enterprises to self-host open-source models.
Cost dynamics are evolving quickly, forcing strategy updates: Open-source developments, efficiency gains, and broader competition are driving down model costs (e.g., OpenAI’s token cost has seen a 10x decrease since 2023). Enterprises must weigh open models’ infrastructure and development costs against licensing fees, pointing to use-case-specific adoption rather than wholesale commitment to either approach.
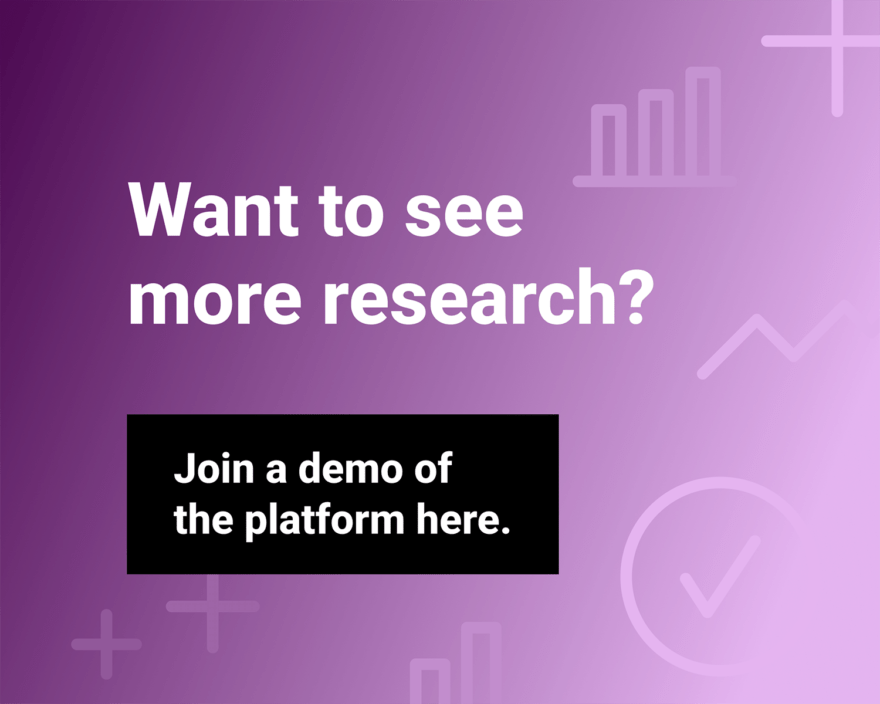