The USA Math Olympiad is an extremely challenging math competition for the top US high school students; the top scorers get prizes and an invitation to the International Math Olympiad. The USAMO was held this year March 19-20. Hours after it was completed, so there could be virtually no chance of data leakage, a team of scientists gave the problems to some of the top large language models, whose mathematical and reasoning abilities have been loudly proclaimed: o3-Mini, o1-Pro, DeepSeek R1, QwQ-32B, Gemini-2.0-Flash-Thinking-Exp, and Claude-3.7-Sonnet-Thinking. The proofs output by all these models were evaluated by experts. The results were dismal: None of the AIs scored higher than 5% overall.
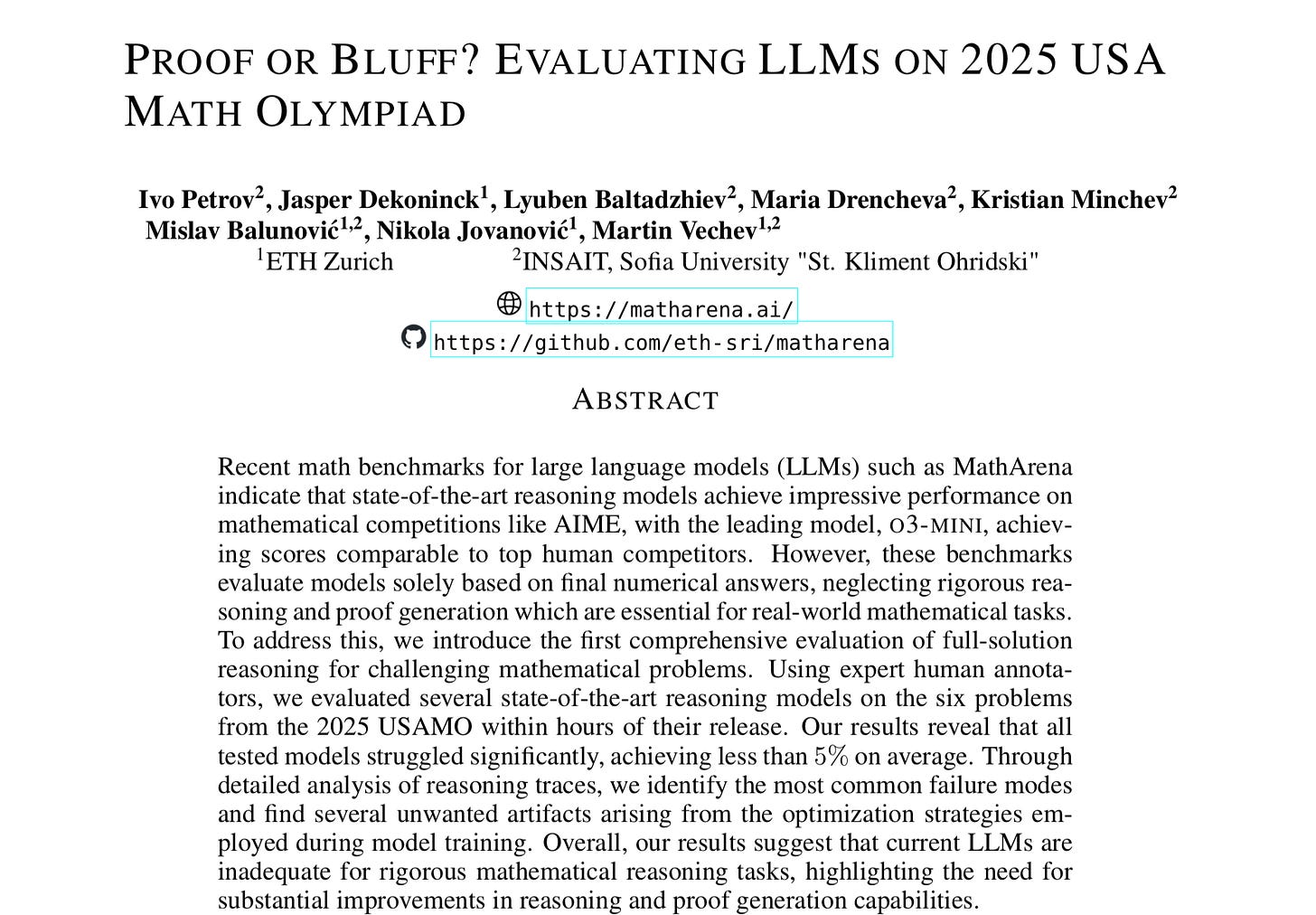
To be sure, a poor showing on the USAMO is not in itself a shameful result. These problems are awfully difficult; many professional research mathematicians have to work hard to find the solution. What matters here is the nature of the failure: the AIs were never able to recognize when they had not solved the problem. In every case, rather than give up, they confidently output a proof that had a large gap or an outright error. To quote the report: “The most frequent failure mode among human participants is the inability to find a correct solution. Typically, human participants have a clear sense of whether they solved a problem correctly. In contrast, all evaluated LLMs consistently claimed to have solved the problems.”
The refusal of these kinds of AI to admit ignorance or incapacity and their obstinate preference for generating incorrect but plausible-looking answers instead are one of their most dangerous characteristics. It is extremely easy for a user to pose a question to an LLM, get what looks like a valid answer, and then trust to it, without doing the careful inspection necessary to check that it is actually right.
If this kind of technology becomes commonly used to answer difficult questions before the problem of generating invalid answers is fixed, we will be in serious trouble. Getting AIs to answer “I don’t know” is one of the most important unsolved challenges facing the field.
Importantly, the neurosymbolic method used by DeepMind’s AlphaProof and AlphaGeometry systems (which we discussed recently) which (more or less) achieved a silver-medal level performance on the 2024 International Math Olympiad, is immune to this problem. AlphaProof and AlphaGeometry generate a completely detailed symbolic proof that can be fed into a formal proof verifier. They can fail to find a proof, but they cannot generate an incorrect proof. But that is because they rely in part on powerful, completely hand-written, symbolic reasoning systems. LLMs are not similarly immune.
§
On X, there have been a couple of reactions to abysmal showing of LLMs, trying to defend LLMs, that are worth discussing. AI researcher Lucas Nestler went so far as to accuse the authors of having faked their results, claiming that o3 did better
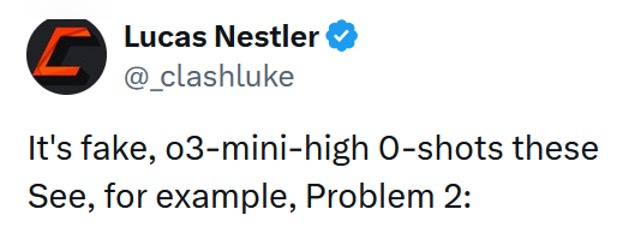
But it turns out that the o3-generated proof of problem 2 that he posted is itself invalid. (The rather technical details, if you’re interested, are included at the end of this essay.)
So Nestler’s experiment does not contradict the finding of the report; it corroborates it. o3, yet again, produced an invalid answer to this problem. It also confirms how dangerous this kind of failing is. The AI output a “proof” that looked plausible to Nestler led Nestler to make a fool of himself in public by outrageously accusing reputable scientists of fakery. (To a trained mathematician, the error in Nestler’s own proof is pretty obvious once pointed out.)
A more serious response was that of Kevin Bryan, who is an economist at U. Toronto:
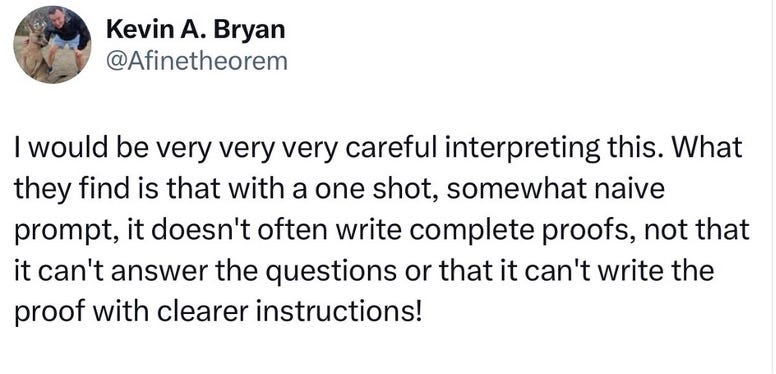
There is certainly some truth to Bryan’s comments. It has often been found that suitable “prompt engineering” can improve the performance of LLMs on reasoning tasks. But there are a few points to be made about this.
First, the burden of proof here is surely on those who would claim that better prompts will get better results. Maybe yes, maybe no; one really wants the evidence. (As the example of Lucas Nestler illustrates, they will have to be careful in evaluating those results.) And importantly, the AIs used in this experiment were already specifically designed by their creators to deal with complex reasoning tasks. (For example, the systems likely already include “hidden prompts” encouraging the AI to work carefully and so on, to the degree that the creators of the AI found useful.) It is by no means certain that additional user prompts will add much,.
Second the “You didn’t say ‘Pretty please’!” sort of excuse that LLM enthusiasts always offer for failures in their favored AIs is only sometimes pertinent. It is – maybe – relevant for judging the usefulness of AIs to regular users of the technology who will take the time to optimize their query style and who are experienced in crafting such prompts. Someone who, day in and day out, is using AI to write code or reports or whatever will take the time to learn what kind of prompts get the best and most reliable results. But what about the hundreds of millions of users who are not specialists in prompting? By now, naïve users expect that, when they ask a question, they can get a reliable answer. Reality is otherwise.
Moreover, however you slice it, this need of the AIs for special cajoling before they will think carefully about questions that are presented to them is a serious weakness, and the demand for special prompting tilts the scale. The high school contestants in the USAMO do not have to be encouraged to think hard about the problems; they are altogether aware that that’s necessary. If indeed the AIs could solve more of these problems with better prompts, then that’s evidence in favor of their mathematical ability but it’s evidence against their ability to judge the right thing to do on their own.
Third and most important: As we said before, the important problem here isn’t that the AIs can’t solve the problems. The important problem is that they sometimes claim to they have solved problems that they have not properly solved; as with hallucinations, this reflects a deeply problematic failure to sanity check their own work.
The really important challenge is not to get the AIs to solve more USAMO problems; it is to get them to say “I give up” when they can’t. And we have yet to see any evidence that any kind of prompt helps in that regard.
Ernest Davis and Gary Marcus are sorry to have to break bad news, once again.
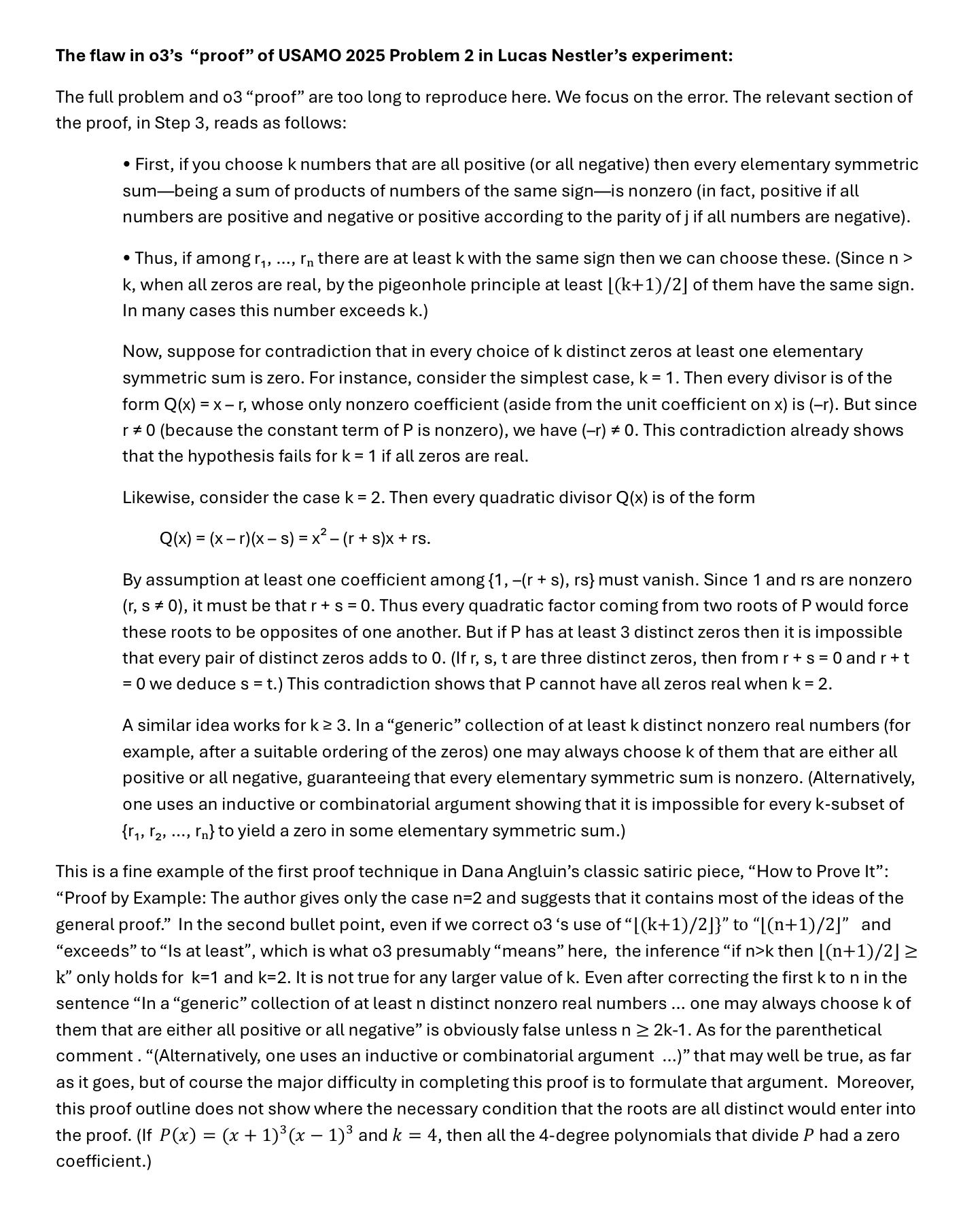