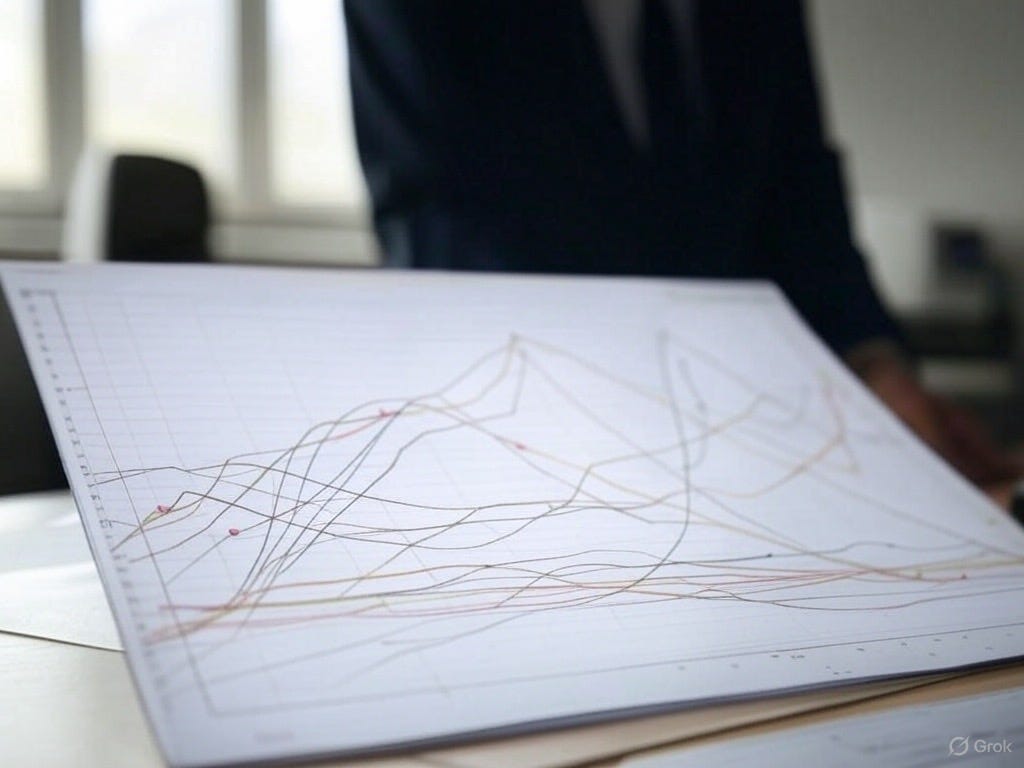
As a scientist, OpenAI’s widely-watched o3 livestream, December 20th, “Day 12 of Shipmas”, which Francois Chollet reported at the time as a breakthough, made me sick to my stomach. I said so at the time, in my essay 𝗼𝟯 “𝗔𝗥𝗖 𝗔𝗚𝗜” 𝗽𝗼𝘀𝘁𝗺𝗼𝗿𝘁𝗲𝗺 𝗺𝗲𝗴𝗮𝘁𝗵𝗿𝗲𝗮𝗱: 𝘄𝗵𝘆 𝘁𝗵𝗶𝗻𝗴𝘀 𝗴𝗼𝘁 𝗵𝗲𝗮𝘁𝗲𝗱, 𝘄𝗵𝗮𝘁 𝘄𝗲𝗻𝘁 𝘄𝗿𝗼𝗻𝗴, 𝗮𝗻𝗱 𝘄𝗵𝗮𝘁 𝗶𝘁 𝗮𝗹𝗹 𝗺𝗲𝗮𝗻𝘀. There were problems with experimental design, misleading graphs that left out competing work, and more.
Later, after I wrote that piece, I discovered that one of their demos, on FrontierMath, was fishy in a different way: OpenAI had privileged access to data their competitors didn’t have, but didn’t acknowledge this. They also (if I recall) failed to disclose their financial contributions in developing the test. And then a couple weeks ago we all saw that current models struggled mightly on the USA Math Olympiad problems that were fresh out of the oven, hence hard to prepare for in advance.
Today I learned that the story is actually even worse than all that: the crown jewel that they reported on the demo — the 75% on Francois Chollet’s ARC test (once called ARC-AGI) doesn’t readily replicate. Mike Knoop from the ARC team reports “We could not get complete data for o3 (high) test due to repeat timeouts. Fewer than half of tasks returned any result exhausting >$50k test budget. We really tried!” The model that is released as “o3 (high)” presumed to be their best model, can’t readily yield whatever was reported in December under the name o3.
The best stable result that ARC team could get from experimenting with the latest batch of publicly-testable OpenAI models was 56% with a different model called o3-medium, still impressive, still useful, but a long way from the surprising 75% that was advertised.
And the lower 56% is not much different from what Jacob Andreas’s lab at MIT got in November. It’s arguably worse; if I followed correctly, and if the measures are the same, Andreas lab’s best score was actually higher, at 61%.
Four months later, OpenAI, with its ever more confusing nomenclature, has released a bunch of models with o3 in the title, but none of them can reliably do what was in the widely viewed and widely discussed December livestream. That’s bad.
Forgive if me I am getting Theranos vibes.
§
Just a couple weeks ago Yafah Edelman at LessWrong reported a related finding, “OpenAI reports that o3-mini with high reasoning and a Python tool receives a 32% on FrontierMath. However, Epoch’s official evaluation[1] received only 11%”; some possible explanations are given, but this is again a very bad look.
And guess what, sometimes o3 apparently cheats, reporting answers that are available on the internet without actually doing the work, as Toby Ord explains in a long thread on X. Essentially Ord argues that o3 is looking up the answer, not computing it.
This in turn is kind of reminiscent of something similar that TransluceAI recently reported last week, in another long thread (too complex to quickly summarize here but worth reading):
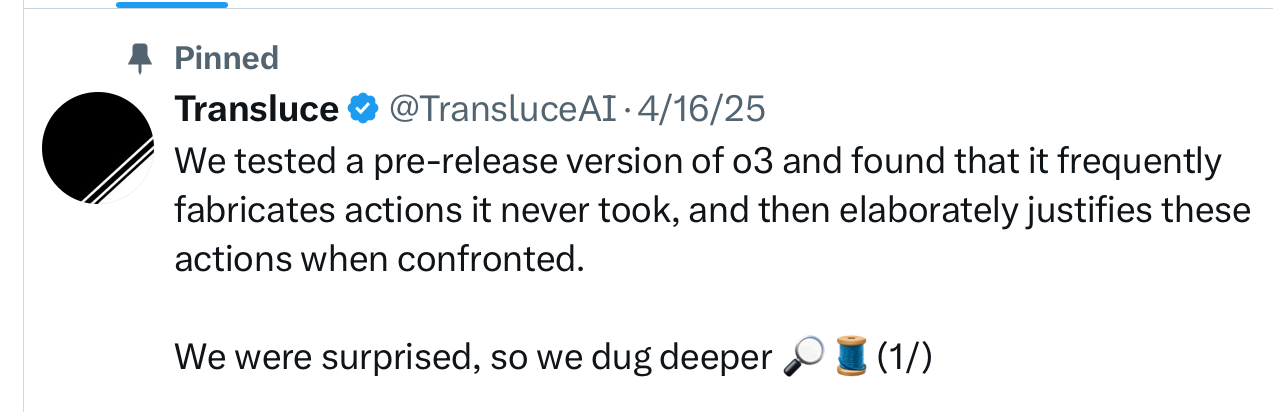
The truth is that we don’t really know how good o3 is or isn’t, and nobody should ever take OpenAI’s video presentations particularly seriously again, until they have been fully vetted by the community. The fact that their flashy result on ARC couldn’t readily be replicated speaks volumes.
§
My trust in OpenAI has never been high; at this point it is extremely low.
And given that Meta also appears to have just juiced some benchmarks, the whole thing is starting to look like a bunch of over-promisers scrambling to make things look better than they really are.
Dr Gary Marcus, Professor Emeritus at NYU, has done enough article reviewing in his career to know when people are trying to pull a fast one.