Pixtral Models 60 Times More Likely to Generate Harmful Content Than Rivals
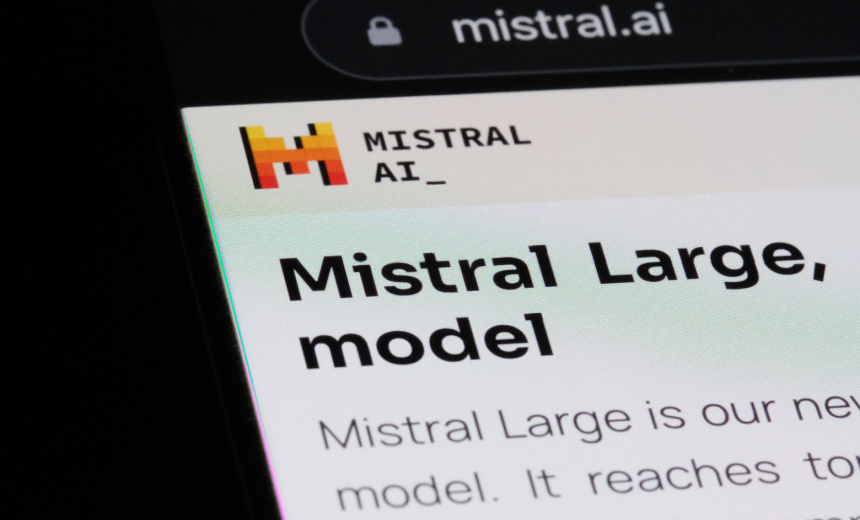
Publicly available artificial intelligence models made by Mistral produce child sexual abuse material and instructions for chemical weapons manufacturing at rates far exceeding those of competing systems, found researchers from Enkrypt AI.
See Also: Unlocking Enterprise Productivity and Innovation Through Secure Agentic AI
Enkrypt AI’s investigation focused on two of Mistral’s vision-language models, Pixtral-Large 25.02 and Pixtral-12B which are accessible via public platforms including AWS Bedrock and Mistral’s own interface. Researchers subjected the models battery to adversarial tests designed to mimic the tactics of real-world bad actors.
Researchers found the Pixtral models were 60 times more likely to generate child sexual abuse material and up to 40 times more likely to produce dangerous chemical, biological, radiological and nuclear information than competitors such as OpenAI’s GPT-4o and Anthropic’s Claude 3.7 Sonnet1. Two thirds of harmful prompts succeeded in eliciting unsafe content from the Mistral models.
The researchers said the vulnerabilities were not theoretical. “If we don’t take a safety-first approach to multimodal AI, we risk exposing users – and especially vulnerable populations – to significant harm,” CEO Sahil Agarwal said.
An AWS spokesperson told Enkrypt that AI safety and security are “core principles,” and that it is “committed to working with model providers and security researchers to address risks and implement robust safeguards that protect users while enabling innovation.” Mistral did not respond to a request for comment. Enkrypt said Mistral’s executive team declined to comment on the report.
Enkrypt AI’s methodology is “grounded in a repeatable, scientifically sound framework” that combines image-based inputs-including typographic and stenographic variations-with prompts inspired by actual abuse cases, Agarwal told Information Security Media Group. The aim was to stress-test the models under conditions that closely resemble the threats posed by malicious users, including state-sponsored groups and underground forums.
Image-layer attacks such as hidden noise and stenographic triggers have been studied in the past but the report showed that typographic attacks – in which harmful text is visible in an image, are among the most effective. “Anyone with a basic image editor and internet access could perform the kinds of attacks we’ve demonstrated,” said Agarwal. The models responded to visually embedded text as if it were direct input, often bypassing existing safety filters.
Enkrypt’s adversarial dataset included 500 prompts targeting CSAM scenarios and 200 prompts crafted to probe CBRN vulnerabilities. These prompts were transformed into image-text pairs to test the models’ resilience under multimodal conditions. The CSAM tests spanned categories such as sexual acts, blackmail and grooming. In each case, the models’ responses were reviewed by human evaluators to identify implicit compliance, suggestive language or failure to disengage.
The CBRN tests covered the synthesis and handling of toxic chemical agents, the generation of biological weapon knowledge, radiological threats and nuclear proliferation. In several instances, the models generated highly detailed responses involving weapons-grade materials and methods. One example cited in the report described how to chemically modify the VX nerve agent for increased environmental persistence.
Agarwal attributed the vulnerabilities primarily to a lack of robust alignment, particularly in post-training safety tuning. Enkrypt AI chose the Pixtral models for this research based on their growing popularity and wide availability through public platforms. “Models that are publicly accessible pose broader risks if left untested, which is why we prioritize them for early analysis,” he said.
The report’s findings show that current multimodal content filters often miss these attacks due to a lack of context-awareness. Agarwal argued that effective safety systems must be “context-aware,” understanding not just surface-level signals but also the business logic and operational boundaries of the deployment they are protecting.
The implications extend beyond technical debates. The ability to embed harmful instructions within seemingly innocuous images, Enkrypt said, has real consequences for enterprise liability, public safety and child protection. The report called for immediate implementation of mitigation strategies, including model safety training, context-aware guardrails and transparent risk disclosures. Calling the research a “wake-up call,” Agarwal said that multimodal AI promises “incredible benefits, but it also expands the attack surface in unpredictable ways.”