Artificial Intelligence (AI) is increasingly making its way into banks’ internal workflows, with chatbots emerging as one of the most common use cases to enhance customer service.
Fifth Third is among the traditional banks leveraging AI via its chatbot, Jeanie, to manage customer inquiries and enhance service quality and satisfaction.
From the AI that fuels Jeanie to the team behind the scenes, we explore how the chatbot came to life — and where it’s headed next.
Fifth Third’s Covid-born chatbot, Jeanie
It started with a question inside the bank’s team: Why not build our own chatbot? From that spark, a homegrown solution took shape — one that now powers conversations behind the scenes while handling customer queries on the front lines.
Jeanie’s inception: Jeanie’s official debut signaled a new chapter in the bank’s approach to chatbot technology.
“We were piloting the concept of a chatbot before Covid started, around 2019-2020,” says Michelle Grimm, Senior Director, Conversational AI at Fifth Third.
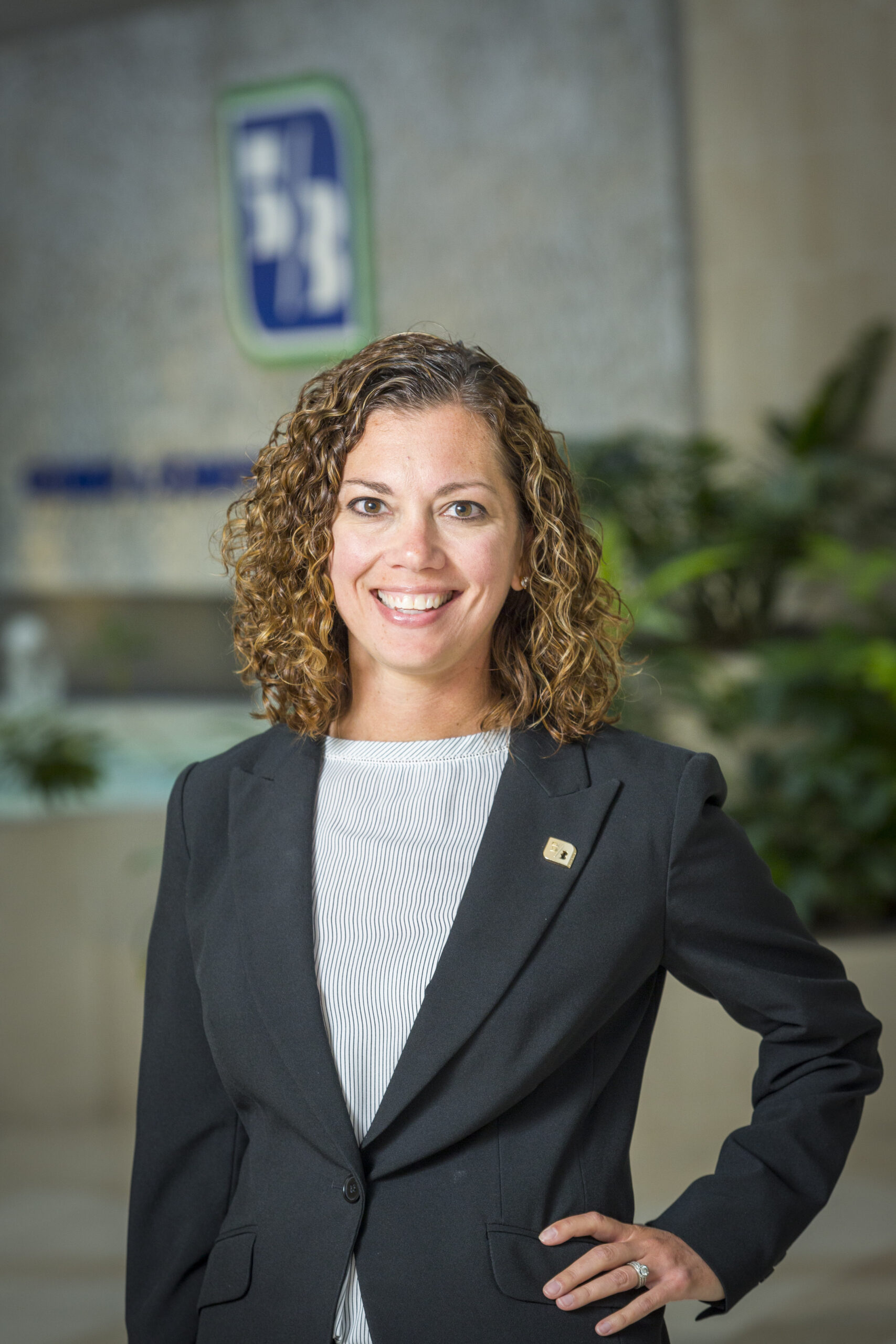
“We started exploring the opportunity of how we could use this from a customer service standpoint. Then Covid happened, and we realized we had to quickly help our call center, which was overwhelmed with phone calls.”
The bank realized that meeting the growing volume of customer inquiries was becoming a challenge, prompting it to fast-track the launch of the pilot program, which was initially tested with just 1% of its customer base. The solution aimed to improve the customer service experience, but also to support agents, who could manage multiple chat conversations at once — something that was not possible with one-on-one phone calls.
“We launched Jeanie faster than we normally would have due to the urgency of the situation,” says Grimm.
Once the initial turbulence of Covid settled, the bank’s team took time to step back and rework Jeanie’s capabilities. They zeroed in on enhancing the Natural Language Understanding (NLU) model. According to Grimm, Jeanie initially picked up on just 20% of customer intents correctly. By reconfiguring the model and developing a proprietary model, they pushed that figure up to about 87%.
“We utilized a more complex nested structure from an NLU perspective, and the model continues to evolve further, and we expect to improve upon what is already a best-in-class model,” she says.
How Jeanie works: Fifth Third currently uses traditional AI to run Jeanie.
“We are using what I would call a traditional AI [for Jeanie],’’ says Grimm. “At this time, we are not using generative AI, but that doesn’t mean in the future we won’t explore it as that technology evolves.”
The bank uses a traditional NLU built on traditional topic modeling to figure out what the customer wants to do. When a customer types in their request, the NLU identifies the intent behind it, classifies the action the customer wants to take, and the bank then verifies that understanding.
For instance, if a customer wants to order a new debit card, the system guides them through a pre-determined flow created by human designers and engineers. Grimm asserts, “It’s a traditional conversational structure that the bot follows.”
On Repeat: Train, test, monitor, refine
Grimm notes that “the process is definitely rigorous” when it comes to testing Jeanie.
It involves two critical teams: data scientists who test and fine-tune the NLU, and quality engineers who ensure the technology works as expected. The team reviews whether Jeanie’s responses align with what’s expected and adjusts training data when things go off-script.
“We constantly evaluate how well the model recognizes customer intents and tweak it as necessary,” she notes. “We look at whether the responses match the expected results and adjust the training phrases if needed.”
The quality engineers ensure the technology aligns with the firm’s flow diagrams and works as intended in the development environment before moving to production. After quality engineering, the flows undergo user acceptance testing to confirm they work as intended.
Human conversations are often complex, with overlapping questions and mixed intentions, which is why the model is continuously refined to better manage real-world interactions.
“We often find that customers interpret questions differently than expected, so we adjust the flows accordingly,” notes Grimm.
“Even after going live, we continuously monitor and refine the chatbot to ensure it meets customer expectations. We have multiple steps from development to production and post-production evaluation to improve the experience.”
Since Jeanie runs on traditional AI, it avoids the risk of hallucinations or generating false information — a common concern with generative AI systems.
Coming soon to Jeanie…
Grimm says the bank handles customer inquiries by answering questions directly, directing users in the app, or routing them to an agent for assistance when necessary. Reflecting on the ongoing evolution of Jeanie, she emphasizes that the bank is constantly scanning for ways to push its capabilities forward.
Fifth Third is focused on evolving Jeanie in several key areas, including:
– Agentic AI: Incorporating agentic AI to strengthen customer service is one of the future paths under consideration. “When I talk about Agentic AI, the possibilities are vast,” Grimm says.
– Task Automation: Moving Jeanie beyond simply guiding users through self-service options to actually completing tasks for them — for example, instead of just showing someone how to transfer money, Jeanie could execute the transaction on their behalf.
– Multilingual Support: Expanding Jeanie’s capabilities to serve customers in multiple languages. Right now, support is only available in English, but the bank aims to open the door to more inclusive service by offering help in additional languages.
Spanish language recognition is a major focus. “We know there’s a significant Spanish-speaking population, especially within our contact center, where we have Spanish-speaking agents,” says Grimm.
Initially, the idea is for Jeanie to spot when a customer prefers Spanish and hand them off to a human. Eventually, the vision is for Jeanie to manage Spanish interactions directly.
– Growing curiosity around voice integration & image generation: While the bank hasn’t committed to voice integration yet, “it’s something we’re interested in,” shares Grimm. On the topic of image generation, Grimm says the firm is keeping an eye on future opportunities, always considering how to make new features work within its vendor-hosted solution.
“These are some of the exciting developments we are exploring for the future,” she says.
Using feedback to inform next steps
The bank gathers feedback from both within the organization and from customers in several ways to sharpen Jeanie’s capabilities:
The bank uses post-interaction surveys to learn whether customers’ problems were effectively addressed after speaking with an agent.
The bank also analyzes customers’ first utterances when engaging with Jeanie, especially when new products or features are released. “This helps us identify if we need to enhance capabilities related to those new offerings,” says Grimm.
Beyond surveys, the Conversational AI team stays in sync with the product and customer experience teams, gathering feedback directly from frontline agents on what could be improved.
Connecting the dots between Jeanie and the VoC program
Fifth Third has enhanced its Voice of the Customer (VoC) program by transitioning from traditional customer surveys to advanced speech analytics. The bank tapped into a third-party analytics tool, NICE Nexidia Analytics and Enlighten AI, enabling the analysis of every customer call across various departments, including consumer banking, collections, and commercial services.
“Our analytics program offers product and process analysis to aid Product, CX, and Lines of Business (LOBs) with customer data, helping identify opportunities or gain feedback on recently implemented changes.”
Instead of sampling a few calls per agent, every conversation gets a sentiment score, allowing the bank to spot trends and opportunities and gain a full, detailed picture of agent and customer experience. This practice allows Fifth Third to capture real-time customer sentiment and behavior, facilitating more effective coaching and training for agents.
“This approach prevents one bad call from disproportionately affecting an agent’s overall score and helps identify areas for improvement,” notes Grimm. “This comprehensive feedback system is a significant win for us.”
Speech analytics was rolled out to all agents in fall 2021, following a pilot phase that began around 2019 with select agent groups using Nexidia to validate the use case.
The cross-over between Jeanie and the VoC program: Can insights from Voice of the Customer programs have the potential to inform functional upgrades to Jeanie?
“I think so,” affirms Grimm.
Here’s how it goes: The VoC program provides customer behavior insights → the bank identifies service gaps Jeanie can fill → it improves Jeanie’s use and visibility based on that feedback.
Insights from the VoC program also enable the bank to identify common customer needs and redirect inquiries that can be addressed digitally.
For example, earlier this year, the team added a prompt to the Interactive Voice Response (IVR) and phone system informing customers, “Did you know Jeanie is open 24/7?” Unlike the phone channel, which has limited service hours, the messaging channel with Jeanie is available around the clock. By placing this message early in the IVR journey, the bank has been able to divert about 700 calls per day to the messaging platform.
Grimm adds that if a customer encounters an error message, the bank now offers an option to connect with Jeanie, rather than simply directing them to a phone number as it had in the past.
“These kinds of efforts not only raise awareness but also guide smarter digital enhancements, steering customers toward channels they prefer to use,” she notes.
The outcome is stronger chatbot engagement and a noticeable uplift in the customer experience.
[Sidebar]: The impact of speech analytics on Fifth Third’s VoC Program
As we talked about the VoC program’s advanced speech analytics, I asked Michelle to share real-world cases that reveal how the team determines when an issue warrants action, and the criteria they use to make that call.
Q: Are the analyzed calls limited to customer service centers, or do they include other types as well?
Michelle Grimm: We’re analyzing all calls coming into our consumer contact center, commercial support center, retail direct sales, customer solutions, and dispute resolution departments. Every single call is scored with multiple algorithms and analyzed, providing aggregate numbers over the month.
We mostly receive inbound customer service calls, but outbound calls make up the majority of contacts for two call centers: Customer Solutions and RDS.
The analysis includes multiple algorithms:
The first being customer sentiment, which is predictive of what a customer would rate in an after-call survey.
The second set of algorithms is enlightened behaviors, focused on specific pillars of customer service, like an agent offering empathy or taking ownership.
Customer sentiment and individual Enlighten behavior algorithms all receive scores on each call, helping call center leadership be more effective coaches. These factors help us understand and improve customer interactions.
Q: How accurate is the AI-driven speech analytics in interpreting customer sentiment?
Michelle Grimm: We found that customer sentiment scores align closely with the ratings customers would likely give in an after-call survey. In a test, supervisors blindly scored calls from A to F based on customer satisfaction, and these scores directly correlated with the actual sentiment scores from a sample of 100 calls. When we had customer surveys, there was also a direct correlation between sentiment scores and survey ratings.
Q: What criteria does the internal team use to decide when to take action on a particular area or service? Is it driven by a high volume of calls regarding the same issue?
Michelle Grimm: Yes, one example I recall involves a situation from a few years ago. We had implemented a digital service that caused a pain point for customers. Despite the digital and security teams insisting that the implementation was functioning correctly, customers were calling in with complaints.
Using speech analytics, we identified a significant number of calls where customers mentioned a specific error message, “Oops,” when logging in digitally. This unique phrasing allowed us to track the increase in calls related to this issue. We documented the number of affected customers and presented this data to our digital teams, highlighting that it was indeed a customer issue. Without speech analytics, we wouldn’t have been able to quantify the problem or bring it to the attention of the digital teams for resolution.
Another recent example from March showed historically high call volumes in the auto loan queue. We used speech analytics to identify the root causes. AI-generated customer call intents were analyzed to see how different topics had changed in volume, helping us discover the drivers behind the increased call volumes. We exported call transcripts and ran them in bulk through AI (Copilot) to identify common issues and concerns for deeper investigation.